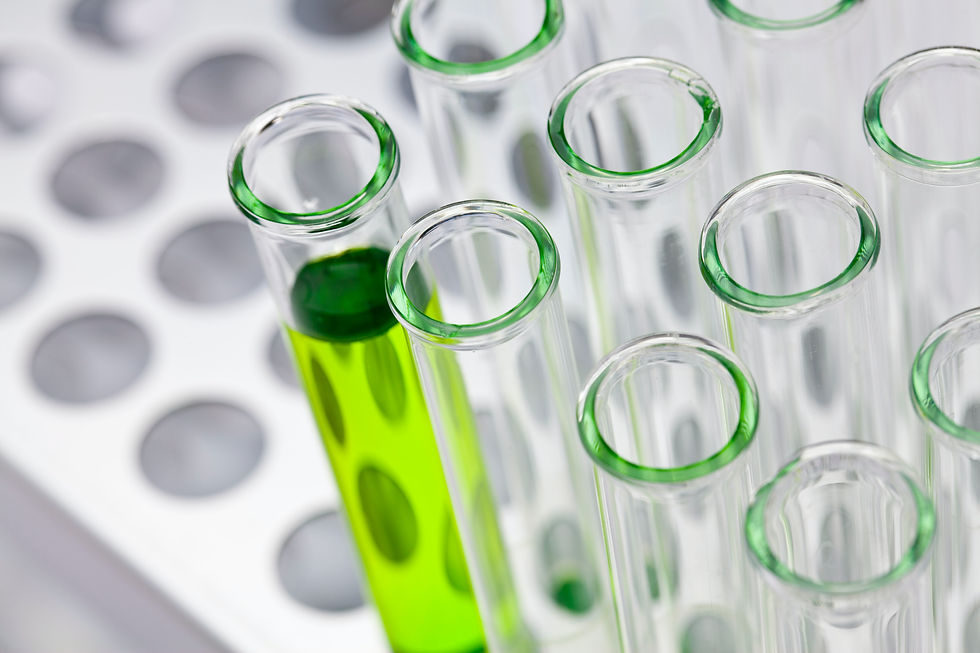
In the ever-evolving world of research, data is the fuel that drives discovery. However, gathering data from entire populations can be impractical, time-consuming, and even impossible. This is where sampling techniques step in, offering powerful tools to select a representative subset that accurately reflects the larger group. This blog delves into the diverse world of sampling techniques, empowering you to choose the right method for your research endeavors.
Why are sampling techniques important?
Sampling techniques provide numerous benefits for researchers:
Efficiency: They allow for collecting data from a manageable number of individuals, saving time and resources compared to studying the entire population.
Cost-effectiveness: Researching the entire population can be expensive. Sampling techniques offer a cost-effective alternative.
Feasibility: Studying every member of a population might be impractical due to factors like size, location, or accessibility. Sampling techniques offer a feasible solution.
Improved accuracy: By ensuring a representative sample, researchers can draw more accurate conclusions about the population.
Exploring the Sampling Landscape:
The world of sampling techniques boasts diverse methods, each with its unique strengths and applications:
1. Probability Sampling:
Random Sampling: Every member of the population has an equal chance of being selected. This method guarantees representativeness but might be challenging for large or geographically dispersed populations.
Stratified Sampling: The population is divided into subgroups (strata) based on pre-defined characteristics (e.g., age, gender, income). A random sample is then drawn from each stratum, ensuring representation of all relevant groups within the population.
Systematic Sampling: The population is ordered in a list, and then every nth individual is selected, starting from a randomly chosen point. This method is efficient but requires a well-ordered list and might introduce bias if there are underlying patterns in the list.
Cluster Sampling: The population is divided into naturally occurring groups (clusters), and some clusters are randomly selected. All members within those clusters are then included in the sample. This method is efficient for geographically dispersed populations but might introduce bias if the clusters are not representative of the population.
2. Non-Probability Sampling:
Convenience Sampling: Individuals readily available or easily accessible are selected, leading to potential bias as the sample might not accurately represent the entire population. This method is often used in exploratory research.
Purposive Sampling: Individuals are selected based on specific criteria deemed relevant to the research question. This method ensures the sample aligns with the research objectives but might not be generalizable to the larger population.
Quota Sampling: The sample is chosen to reflect specific characteristics of the population in predetermined proportions. This method can be efficient but relies on accurate knowledge of the population's characteristics.
Snowball Sampling: Individuals recruit others from their network who meet the study criteria. This method can be useful for reaching hidden populations but can be prone to bias if the initial participants are not representative.
Choosing the Right Technique:
The optimal sampling technique depends on several factors:
Research objectives: What are you trying to learn?
Population characteristics: What is the size and nature of the population?
Resource limitations: What are your time and budget constraints?
Ethical considerations: Will the chosen method respect the rights and privacy of participants?
Conclusion:
Effective sampling techniques are like skilled investigators, meticulously selecting a representative group to unveil the truth about the larger population. By understanding the various methods and their strengths and limitations, you can equip yourself with the tools needed to conduct reliable research and draw accurate conclusions, ultimately furthering the quest for knowledge and discovery.
Comments